Getting your Trinity Audio player ready...
|
NVIDIA everywhere
With the exception of cooling technologies, almost every compute instance demonstrated in the show was from NVIDIA.
Walking through the hall, it was hard to miss the multiple GB200 NVL72 racks displayed in numerous booths and the slew of “NVIDIA partner” signs across the hall. The yet-another-record-quarter results that NVIDIA published during the week of the show also contributed to the notion of NVIDIA’s dominance in today’s compute world, and specifically when it comes to AI training and inference infrastructure.
And it is not just around compute GPUs and CPUs. The supporting infrastructure – like storage, networking, and even power and cooling – is also provided by or certified by (or at least aligned with the blueprint of) Nvidia.
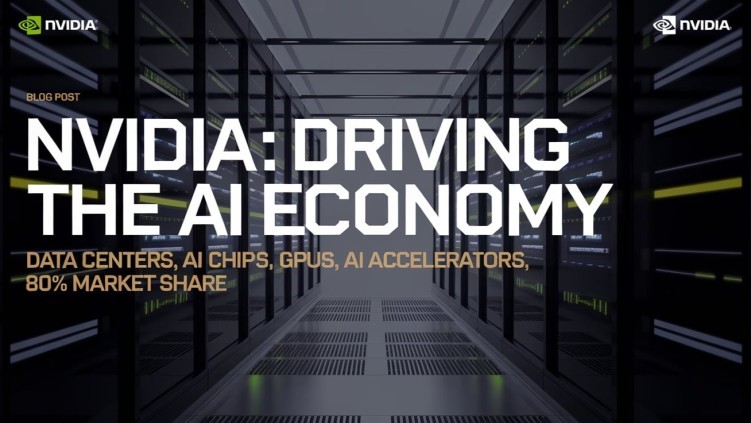
Working with NVIDIA?
So how is it living in the “NVIDIA era?”
During the show, I managed to talk with many customers and partners that use and sell NVIDIA’s gear, from GPUs to SmartNICs. The common response I heard was one of ambivalence.
On the one hand, having NVIDIA as a de facto industry standard makes life pretty easy. A lot of integration headaches are avoided when you stick to a well-defined reference architecture.
On the other hand, such a dominant vendor almost eliminates control over your architecture, and leaves you with no leverage, whatsoever, with regard to pricing, architecture and supply chain. This is true for both end customers and partners/resellers.
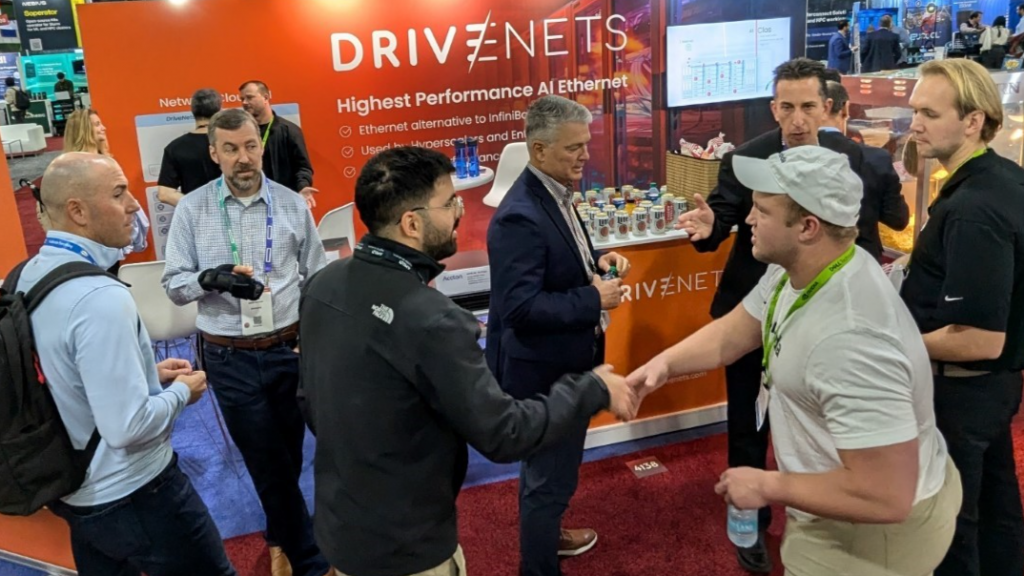
Not working with NVIDIA?
So, what’s the alternative?
When it comes to GPUs, there are not many alternatives to NVIDIA’s ecosystem. Though we are starting to see good reception for the AMD Instinct solution, everyone is assuming that this will not last forever.
In the meanwhile, customers and partners alike are looking for an alternative for NVIDIA’s dominance in the supporting architecture – and in the networking domain, in particular.
Networking first
Why is networking so important? For one, as discussed many times before, it is identified as one of the key points that influence the performance (in terms of job completion time, JCT) of the entire GPU cluster. This is particularly true if it is a very large cluster utilized for training.
Second, this domain has multiple solutions, and not all of them are sufficient in terms of performance (high capacity, low tail latency, low jitter, fast failure recovery). But, and this is an important point, the solution with the highest performance does not come from NVIDIA.
Scheduling and fine-tuning
The key to achieving a high-performance AI backend network is scheduling. This is because the nature of RoCE (RDMA over Converged Ethernet) requires low tail latency, low jitter, and low packet loss. This calls for minimum congestion that can only be mitigated or avoided by a scheduling mechanism.
There are two types of scheduling mechanisms – endpoint scheduling (implemented in InfiniBand, Ultra Ethernet, NVIDIA’s Spectrum-X and others) and fabric scheduling (implemented in DriveNets’ DDC, Meta’s DSF and Arista’s DES).
While endpoint scheduling can yield reasonable performance, the highest performance networking solution uses a fabric-scheduled architecture. Since, at the end of the day, NVIDIA does not provide such a solution, you can run a non-NVIDIA network and benefit. The non-NVIDIA fabric-scheduled solution not only isn’t locked into the NVIDIA ecosystem, but it also offers superior performance.
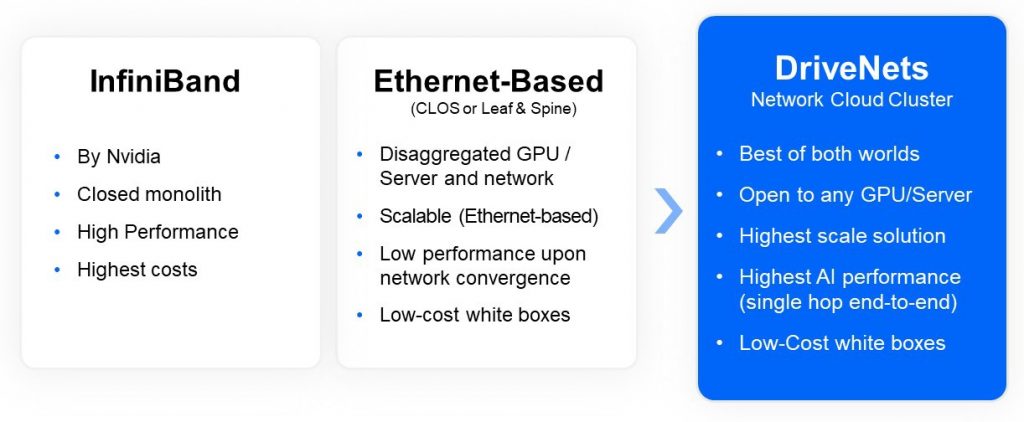
Another positive side effect, which for some customers is most important, is the time-to-deploy benefit. Endpoint scheduled solutions require lots of fine-tuning, both during bring-up and in any case of changing the type of workload running on the GPU cluster. For fabric-scheduled solutions the implementation is much simpler and is, basically, a plug-and-play process, with no required fine-tuning or special knowledge/skill.
From AI networking trials to massive deployments
While networking is not typically the greatest concern of most customers, its effect becomes crucial as AI clusters grow larger. While we heard a lot of customers last week talking about fairly small deployments this year, most of them have plans to deploy much larger clusters in the near future. This makes today a very good time to consider the best networking solution to meet long-term needs.
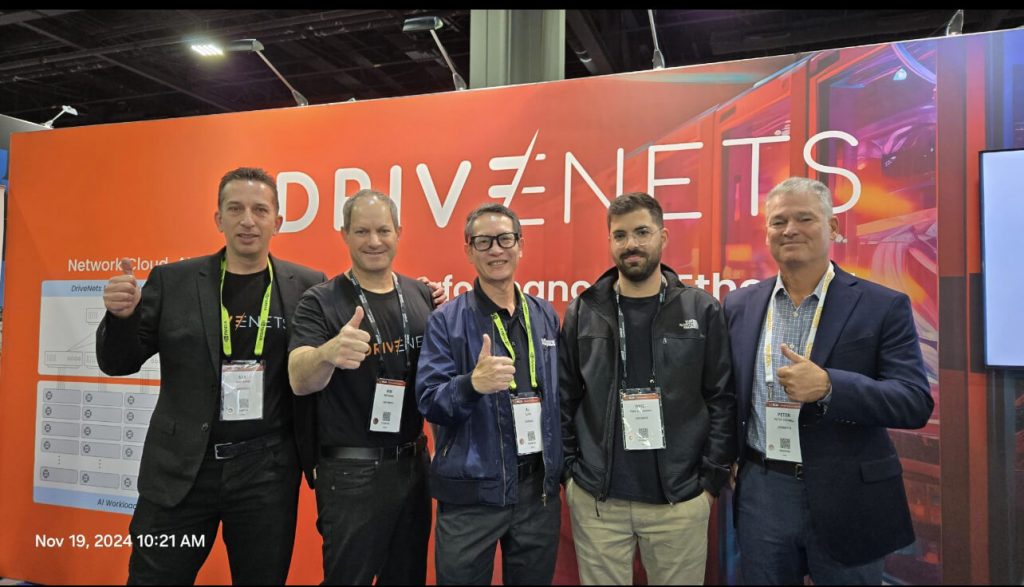
eGuide
AI Cluster Reference Design Guide