Case Study: Uncompromised GPU Performance with Ethernet-based AI Fabric
Challenges – Seeking an Alternative to InfiniBand
The North American research firm has a rich history of using AI and machine learning for research purposes, including deploying neural networks within large-scale HPC workloads to significantly accelerate the simulation process. Until recently, this leading research firm was solely dependent on NVIDIA’s ecosystem. However, growing requirements have led the firm to seek alternative network solutions that can enhance flexibility and vendor diversity while maintaining uncompromised GPU performance.
Seeking an alternative to InfiniBand is not a simple task. InfiniBand delivers great performance, and most Ethernet solutions are unable to deliver on-par job completion time (JCT) results for large-scale AI workloads. However, shifting to Ethernet can allow a simpler cluster deployment, since Ethernet is an industry standard and a known skill for most IT teams, unlike InfiniBand. Additionally, the standard IP protocol offers a significant improvement in flexibility.
Today, for example, having storage and GPU communications under the same fabric with InfiniBand requires the use of IPoIB (IP over InfiniBand), which is less efficient and requires additional configuration and tuning to optimize performance. Another InfiniBand limitation is that scaling across remote locations requires additional gateway devices to bridge the gap between InfiniBand and Ethernet. Overall, adopting a more open Ethernet-based solution unlocks new capabilities, including support for long distance AI workloads, standardization, improved
The complete requirements for the new AI fabric solution were:
- High-performance at scale: making uncompromised GPU performance a top priority given target cluster size of 2K GPUs.
- Enhanced observability: leveraging Prometheus toolkit for advanced application and network monitoring, as well as more comprehensive data analysis and visualization
- Plug-n-play setup: minimizing the need for continuous network fine-tuning
- Long-distance AI workloads: supporting AI clusters across several geographical locations
- Standard Ethernet-based solution: enabling simpler cluster deployment and a unified fabric for storage and GPUs
- Support and R&D attention: enhancing support, bug fixes, and feature enhancements (as enterprise companies struggle for NVIDIA’s attention)
SOLUTION: Ethernet-based AI Fabric
The DriveNets Network Cloud-AI solution and its scheduled fabric met all of the research firm’s requirements. During proof-of-concept testing, the solution delivered up to 30% better performance than other Ethernet-based options and performance on par with InfiniBand, all without extensive fine-tuning. The solution’s scheduled fabric minimized congestion and jitter, and its predictable connectivity offered consistent low latency and nanosecond failover recovery.
Key features
DriveNets Network Cloud-AI offer a holistic solution based on distributed scheduled fabric architecture:
- Clos Topology Offers a scalable design where top-of-rack (ToR) switches serve as network cloud packet forwarders (NCPs), while fabric switches act as network cloud fabrics (NCFs)
- Scheduled Ethernet Fabric Ensuring the entire cluster, even with thousands of GPUs, functions as a single Ethernet node by utilizing cell sparing and end-to-end virtual output queueing (VOQ)
- Open & Standardized Architecture Ensuring compatibility with any NIC, GPU and optics, thereby supporting vendor
diversity and cost efficiency
Deployment details
- Initial setup: 864 NVIDIA Blackwell GPUs, with expected scale to 2K GPUs
- Hardware elements: a cluster of 32 NCPs (ToR switches) and 10 NCFs (fabric switches) using NCP5 and
NCF2 white boxes by Accton, powered by Broadcom Jericho3AI and Ramon3 chipsets - Software: DriveNets Network Cloud software orchestrating the cluster
In addition to the AI fabric, a data center interconnect (DCI) powered by DriveNets Network Cloud was deployed, connecting the cluster to a nearby campus with the addition of eight NCPs.
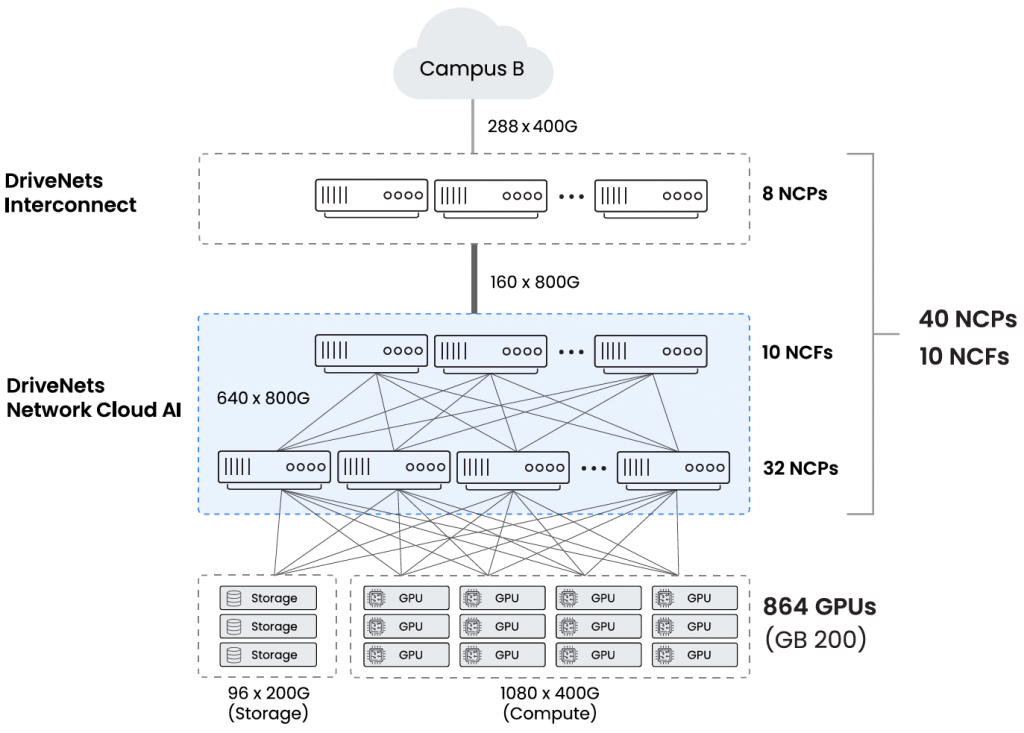
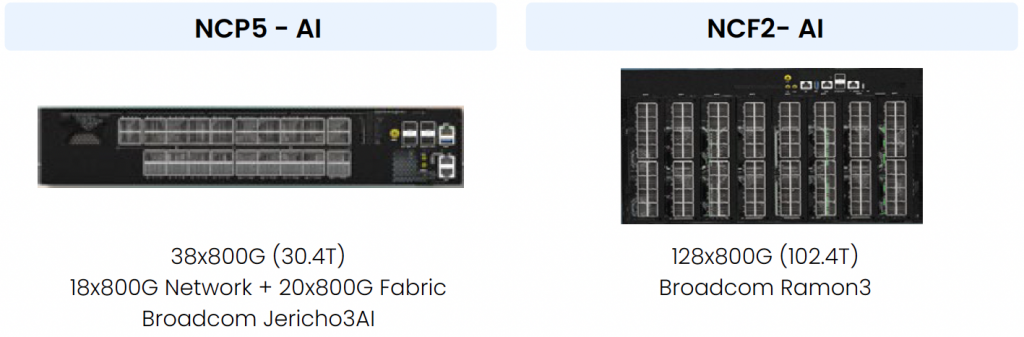
RESULTS
The research firm achieved its performance and operational goals with DriveNets Network Cloud-AI, realizing the following benefits:
- High GPU utilization:: validated job completion time (JCT) improvements over other Ethernet solutions and on par with InfiniBand
- Plug-n-play deployment:: high performance from day one, with minimal fine-tuning
- Open architecture:: Sbuilt on widely recognized Ethernet protocol and compatible with any NIC, GPU, and optics hardware component
- Enhanced vendor support: DriveNets’ vast experience and close support enabled rapid bug fixes and the development of tailored solutions requested by the research firm —solutions that were not offered by other vendors (e.g., support of Prometheus toolkit).
“We enjoy this relationship. We enjoy the disruption. We enjoy the ability to work together and push some boundaries in our ability to deliver not just software, but actually a full service. We can deploy.”
Senior Network Executive, Global Tier-1 Operator in North America
SUMMARY
Like many other research companies, this U.S.-based firm has a strong legacy of using AI and ML in its research. Until recently, the firm’s AI workloads relied exclusively on NVIDIA’s InfiniBand solution. However, growing requirements pushed the firm to search for a more flexible and open solution without compromising GPU utilization.
DriveNets Network Cloud-AI emerged as a compelling alternative, offering Ethernet-based high-performance networking. The DriveNets solution not only met the firm’s top requirement of high performance at scale, but also simplified deployment, enhanced observability, and provided greater vendor flexibility. By adopting DriveNets, the company achieved high GPU utilization while reducing operational costs and increasing operational flexibility.