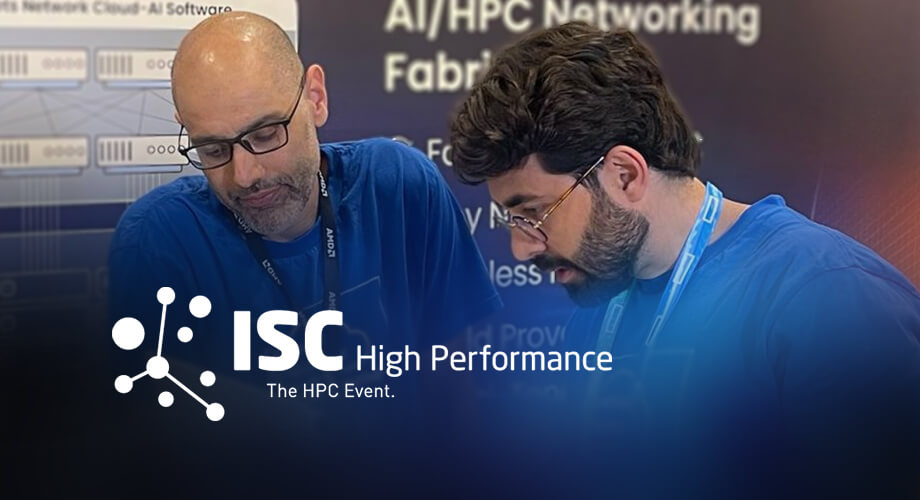
AI Networking
Reading Time: 4 minutes
I just got back from rainy Hamburg after another great ISC conference. ISC 2025 was an event full of energy...
Read moreAI Networking
Reading Time: 4 minutes
I just got back from rainy Hamburg after another great ISC conference. ISC 2025 was an event full of energy...
Read moreAI Networking
Reading Time: 4 minutes
Parallelism is the engine behind modern AI scalability, but it’s only as effective as the networking infrastructure supporting it. The...
Read moreAI Networking
Reading Time: 4 minutes
Today high-performance computing is no longer confined to hyperscalers. NeoClouds and Enterprises across finance, healthcare, telecom, and other industries are...
Read moreAI Networking
Reading Time: 4 minutes
As artificial intelligence (AI) workloads scale to thousands—and sometimes hundreds of thousands—of GPUs, the underlying network architecture becomes incredibly important....
Read moreAI Networking
Reading Time: 5 minutes
Prized for its low latency and lossless transport, InfiniBand has been for years the go-to networking technology for high-performance computing...
Read moreSign up to get the latest news and insights.
Service Provider
Reading Time: 4 minutes
AIOps (artificial intelligence for operations) means using artificial intelligence (AI) and machine learning (ML) to make network operations smarter and...
Read moreNetwork Infrastructure
Reading Time: 4 minutes
First off, may the force be with you on this May the Fourth. The most oft-repeated line, “May the Force be...
Read moreOperations
Reading Time: 5 minutes
Imagine never having to schedule a 3 AM network maintenance window again. No late-night cutovers, no telling customers “service will...
Read moreService Provider
Reading Time: 8 minutes
At DriveNets’ MWC25 panel, executives from AT&T, Orange, KDDI, Cox Communications, and Broadcom came together to discuss how disaggregation, convergence,...
Read moreCable
Reading Time: 3 minutes
It’s been an interesting week here in Denver, as the Cable Next-Gen Technologies & Strategies event provided an insightful look...
Read moreAI Networking
Reading Time: 4 minutes
It was an exhausting week here in San Jose at Nvidia GTC 2025. The conference is definitely becoming the main...
Read moreAI Networking
Reading Time: 5 minutes
Good Today but Better Tomorrow with Fabric-Scheduled Ethernet As data center demands grow exponentially, enterprises and cloud providers alike are...
Read moreService Provider
Reading Time: 3 minutes
Typically, I seek out statistical data to validate technological statements. But this time, it’s obvious. Everyone is experiencing the surge...
Read moreService Provider
Reading Time: 3 minutes
Thinking about disaggregation takes me back to late nights in the network lab at Orange in 2008. I particularly remember...
Read moreAI Networking
Reading Time: 4 minutes
If it were up to my twins, they would spend all their waking hours on their smartphones. That’s why I...
Read moreService Provider
Reading Time: 4 minutes
February is always a great month to review the state of the telecom industry. And Barcelona is a great place...
Read moreAI Networking
Reading Time: 3 minutes
In today’s competitive artificial intelligence (AI) landscape, hyperscalers and large enterprises are rapidly recognizing the critical need for open, scalable,...
Read moreAI Networking
Reading Time: 4 minutes
An artificial intelligence (AI) cluster architecture integrates backend compute and storage networking components to meet the demands of high-performance AI...
Read moreAI Networking
Reading Time: 2 minutes
Drug development/discovery and biotech are R&D-intensive life sciences fields in which the analysis of very large datasets is key. From...
Read moreAI Networking
Reading Time: 4 minutes
A new breed of cloud provider has emerged – the “NeoCloud” provider. These companies, like CoreWeave, Crusoe, and Lambda, focus...
Read moreAI Networking
Reading Time: 3 minutes
2024 was an interesting year. Now let’s look at what experts from the industry are saying about networking in the...
Read moreAI Networking
Reading Time: 2 minutes
The automotive industry has changed, in the last decade, to become a software-centric, computation-intensive industry. The drive towards higher and...
Read moreAI Networking
Reading Time: 2 minutes
The finance sector is perhaps the one with the largest number of artificial intelligence (AI) / machine learning (ML) use...
Read moreAI Networking
Reading Time: 4 minutes
The end of each year is an important time to reflect on accomplishments, as well as challenges. The last 12...
Read moreIndustry Outlook
Reading Time: 2 minutes
As we reach the end of the year and get deeper into the holiday spirit, we’ve given our spin on...
Read moreAI Networking
Reading Time: 2 minutes
Yes, it’s that season of the year, again – predictions time. We’ve learned a lot about AI infrastructure during the last...
Read moreAI Networking
Reading Time: 8 minutes
While latency is often discussed as an unavoidable bottleneck in networking and system performance, advancements in technology are changing this...
Read moreAI Networking
Reading Time: 4 minutes
Alternatives for your AI Networking fabric Just got back from Atlanta, this year’s site of the SC24 conference. Here are some...
Read moreDisaggregation
Reading Time: 4 minutes
One of DriveNets’ main missions is solving the key challenges of service providers (SPs) – declining revenues and rising network...
Read moreNetwork Infrastructure
Reading Time: 5 minutes
A network isn’t some monolithic black box. It’s a set of components that cooperate to provide connectivity that’s both economical...
Read more